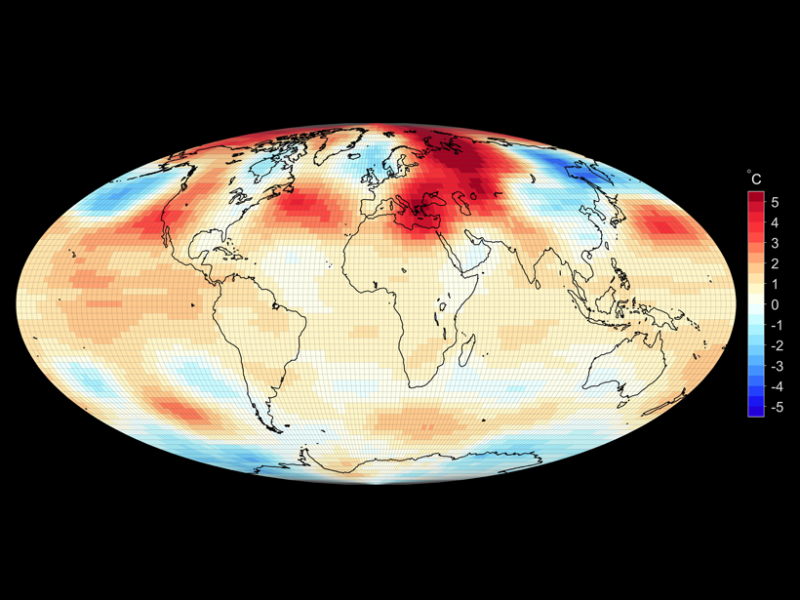
Temperature anomalies (deviations from the 1981–2010 monthly mean in degrees Celsius) estimated from advanced microwave sounding unit data for February 2016. Estimates of their spatially averaged magnitude, using sum-of-squares-based average-deviation measures, such as the root-mean-square or standard deviation, would erratically overestimate their true spatially averaged magnitude. (Data are from the National Space Science and Technology Center, University of Alabama in Huntsville.)
Even as Category 5 Hurricane Irma churns toward South Florida (on its present track) there is renewed interest in the level of accuracy of climate change related forecasts. This is especially in terms of whether the intensity and frequency of major storms is increasing. I already dealt with a number of these issues in my Sept. 2nd post to do with climate troll Roger Pielke Jr.'s obfuscations. This post concerns advances in the statistics determining predictive accuracy for climate models, and how a new investigation discloses they may be more accurate than originally reported.
The new research, published by Cort J. Willmott, Scott M. Robeson and Kenji Matsuura shows that in fact our climate models may well have been more - not less -accurate than expected. (Eos: Earth & Space Science News, September, p. 13).
As the authors note, all sciences use models to predict physical phenomena but the predictions are only as good as the models on which they are based. For example, in my paper Limitations of Empirical-Statistical Methods of Solar Flare Prognostication’ appearing on pp. 276-284 of the 'Solar Terrestrial Predictions Proceedings- Meudon' e.g.

I noted the limits of prognostication for given (magnetic, optical, radio) observational thresholds in respect of forecasting geo-effective solar flares. These are the flares that can interfere with communications on Earth as well as aircraft navigation controls. The problem is that the models in question were mainly hybrid, combining a lot of statistics with physical aspects, for example accounting for release of magnetic free energy in specific flares using:
¶ / ¶ t {òv B2/2m dV} = 1/m òv div[(v X B) X B] dV
- òv {han | Jms |2 }dV
The limits of climate models are also dependent on statistics - mainly the statistical assessment of errors in model prediction and estimation. See, for example:
http://www.ipcc.ch
for recent IPCC reports that present commonly used estimates of average error to evaluate the accuracies of global climate model simulations. There is also another recently developed model evaluation metrics package to be found at:
http://bit.ly/PCMDI-metrics
which similarly assesses, visualizes and compares model errors. In many ways, this is reminiscent of many of the papers appearing in the aforementioned Solar-Terrestrial Predictions volume, except the latter was focused on solar flares, CMEs and auroral substorms. Another similarity is the climate package evaluated measures for the average differences between observed and predicted values for the root mean square errors (RMSEs, e.g. s[x], s[y] ).
Willmott et al's contribution as noted in their paper is:
"We contend, however, that average error measures based on sums of squares, including the RMSE, erratically overestimate average model error. Here we make the case that using an absolute value–based average-error measure rather than a sum-of-squares-based error measure substantially improves the assessment of model performance."
They go on to note (ibid.):
"Our analyses of sum-of-squares-based average-error measures reveal that most models are more accurate than these measures suggest [Willmott and Matsuura, 2005; Willmott et al., 2009]. We find that the use of alternative average-error measures based on sums of the absolute values of the errors (e.g., the mean absolute error, or MAE) circumvents such error overestimation.
At first glance, the distinction between average-error measures based on squared versus absolute values may appear to be an arcane statistical issue. However, the erratic overestimation inherent within sum-of-squares-based measures of average model estimation error can have important and long-lasting influences on a wide array of decisions and policies. For example, policy makers and scientists who accept the RMSEs and related measures recently reported by the IPCC [Flato et al., 2013] are likely to be underestimating the accuracy of climate models. If they assessed error magnitude–based measures, they could place more confidence in model estimates as a basis for their decisions."
Willmott et al's discovery of the acceptance of RSMEs is for sure a momentous find, and suggests a drastic need for re-assessment of climate model accuracy. As they further explained above, this is not merely an "arcane" or esoteric statistical issue:
Hence, their recommendation needs to be taken seriously by climate modelers, to wit:
"Our recommendation is to evaluate the magnitude (i.e., the absolute value) of each difference between corresponding model-derived and credibly observed values. The sum of these difference magnitudes is then divided by the number of difference magnitudes. The resulting measure is the MAE. In effect, MAE quantifies the average magnitude of the errors in a set of predictions without considering their sign. Similarly, the average variability around a parameter (e.g., the mean) or a function is the sum of the magnitudes of the deviations divided by the number of deviations. This measure is commonly referred to as the mean absolute deviation (MAD)."
The authors most noteworthy conclusion is:
"Although the lower limit of RMSE is MAE, which occurs when all of the errors have the same magnitude, the upper limit of RMSE is a function of both MAE and the sample size (√n × MAE) and is reached when all of the error is contained in a single data value [Willmott and Matsuura, 2005].
An important lesson is that RMSE has no consistent relationship with the average of the error magnitudes, other than having a lower limit of MAE."
Adding:
"we concur with J. S. Armstrong, who after assessing a number of forecast evaluation metrics warned practitioners, “Do not use Root Mean Square Error” [Armstrong, 2001]. Only in rare cases, when the underlying distribution of errors is known or can be reliably assumed, is there some basis for interpreting and comparing RMSE values.
More broadly, comparable critiques can also be leveled at sum-of-squares-based measures of variability, including the standard deviation (s) and standard error [Willmott et al., 2009]. Their roles should be limited to probabilistic assessments, such as estimating the sample standard deviation as a parameter in a Gaussian distribution."
Given the propensity of the climate change denier (and obfuscator) brigade to impugn any climate modeling and the integrity of the models themselves, it is important these more refined error assessment and evaluation procedures be circulated and used.
In many ways the authors' advice in respect of climate model error assessment reminded me of my own advice to flare modelers using a multiple regression format. (say relating geo-effective flare frequency to sunspot area, A, and magnetic class, C). That is, I advised that the optimum forecast accuracy was best approximated by use of the coefficient of multiple determination - or the percentage of total sample variation accounted for by regression on the independent variables (e.g. A,C). I also strongly advised the use of Poisson statistics to ascertain the significance of solar events, including coronal mass ejections.
No comments:
Post a Comment